Technologies change over time at different rates. Here we study the factors that drive technology evolution, and we ask why some technologies improve more quickly than others. We aim to characterize and explain these rates of change, taking approaches that range from the statistical analysis of large datasets to the development of new theory. We have a particular interest in relating the design features of technologies to their rates of improvement. Recent papers have been published in the areas of theory development, statistical analysis of large datasets, decomposition of historical cost trends, and patenting trends.
Theory development: We study a simple model for the evolution of the cost (or more generally the performance) of a technology or production process. The technology can be decomposed into n components, each of which interacts with a cluster of d – 1 other components. Innovation occurs through a series of trial-and-error events, each of which consists of randomly changing the cost of each component in a cluster, and accepting the changes only if the total cost of the cluster is lowered. We show that the relationship between the cost of the whole technology and the number of innovation attempts is asymptotically a power law, matching the functional form often observed for empirical data. The exponent of the power law depends on the intrinsic difficulty of finding better components, and on what we term the design complexity: the more complex the design, the slower the rate of improvement. Letting d as defined above be the connectivity, in the special case in which the connectivity is constant, the design complexity is simply the connectivity. When the connectivity varies, bottlenecks can arise in which a few components limit progress. In this case the design complexity depends on the details of the design. The number of bottlenecks also determines whether progress is steady, or whether there are periods of stasis punctuated by occasional large changes. Our model connects the engineering properties of a design to historical studies of technology improvement.

Figure 1. Comparison of the cost for a simulation of a single realization of the production recipe model (circles) to the predicted expected cost (solid curve), for the case of constant out-degree. n is the number of components and d is the connectivity.
- McNerney J, Farmer JD, Redner S, Trancik JE, Role of Design Complexity in Technology Improvement, Proceedings of the National Academy of Sciences, 2011, Vol. 108, pp. 9008-9013 link.
Statistical analysis of large datasets: Forecasting technological progress is of great interest to engineers, policy makers, and private investors. Several models have been proposed for predicting technological improvement, but how well do these models perform? An early hypothesis made by Theodore Wright in 1936 is that cost decreases as a power law of cumulative production. An alternative hypothesis is Moore’s law, which can be generalized to say that technologies improve exponentially with time. Other alternatives were proposed by Goddard, Sinclair et al., and Nordhaus. These hypotheses have not previously been rigorously tested. Using a new database on the cost and production of 62 different technologies, which is the most expansive of its kind, we test the ability of six different postulated laws to predict future costs. Our approach involves hindcasting and developing a statistical model to rank the performance of the postulated laws. Wright’s law produces the best forecasts, but Moore’s law is not far behind. We discover a previously unobserved regularity that production tends to increase exponentially. A combination of an exponential decrease in cost and an exponential increase in production would make Moore’s law and Wright’s law indistinguishable, as originally pointed out by Sahal. We show for the first time that these regularities are observed in data to such a degree that the performance of these two laws is nearly tied. Our results show that technological progress is forecastable, with the square root of the logarithmic error growing linearly with the forecasting horizon at a typical rate of 2.5% per year. These results have implications for theories of technological change, and assessments of candidate technologies and policies for climate change mitigation.
This data can be explored on the following website: pcdb.santafe.edu.
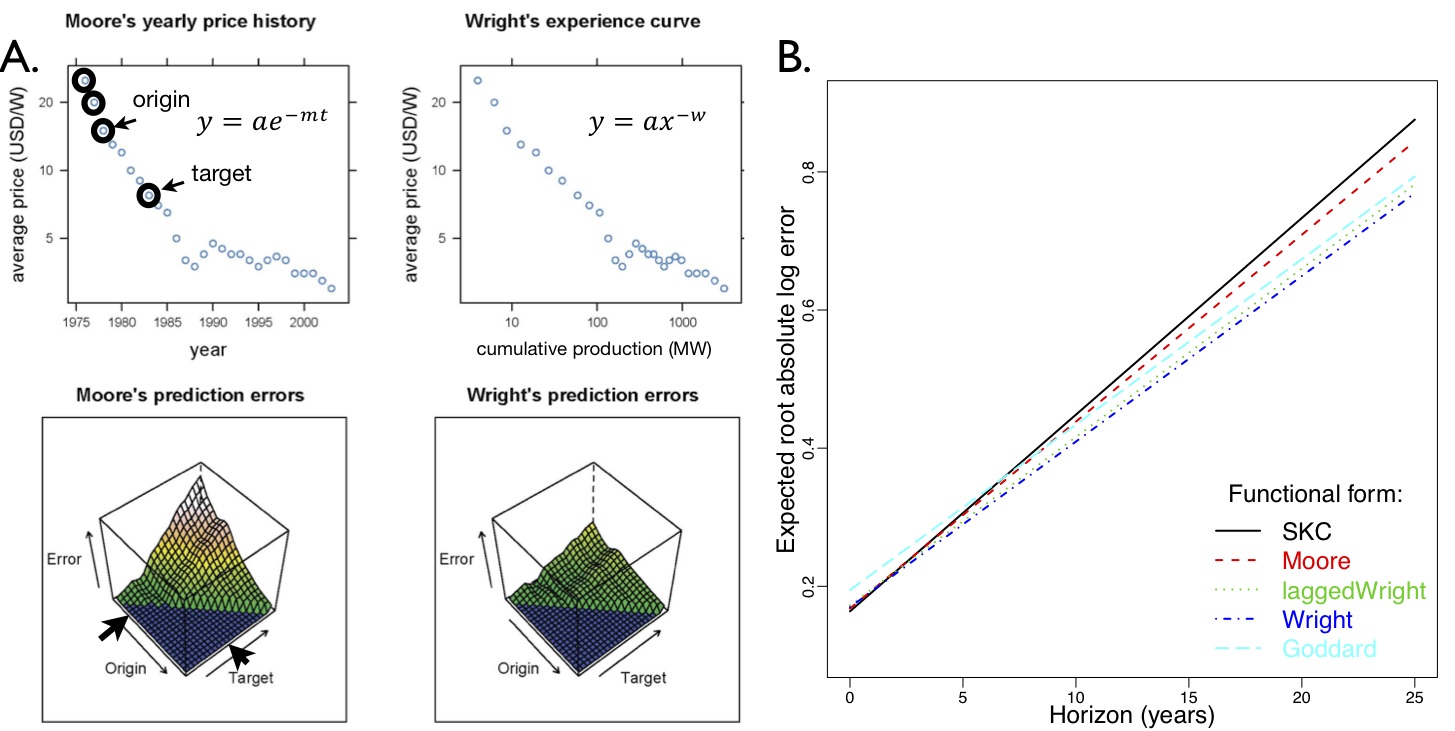
Figure 2. A) Predictions of changes to photovoltaics cost based on time-based and production-based models are compared using hindcasting. B) The performance of several proposed models was compared using a mixed effects statistical model, based on a database of approximately 100 performance curves (energy, chemicals, hardware etc.).
- Nagy B, Farmer JD, Bui QM, Trancik JE, Statistical basis for predicting technological progress, PLoS One, 2013, Vol. 8, e52669 link.
Decomposition of historical cost trends: We study the cost of coal-fired electricity in the United States between 1882 and 2006 by decomposing it in terms of the price of coal, transportation cost, energy density, thermal efficiency, plant construction cost, interest rate, capacity factor, and operations and maintenance cost. The dominant determinants of cost have been the price of coal and plant construction cost. The price of coal appears to fluctuate more or less randomly while the construction cost follows long-term trends, decreasing from 1902 to 1970, increasing from 1970 to 1990, and leveling off since then. Our analysis emphasizes the importance of using long time series and comparing electricity generation technologies using decomposed total costs, rather than costs of single components like capital. By taking this approach we find that the history of coal-fired electricity suggests there is a fluctuating floor to its future costs, which is determined by coal prices. Even if construction costs resumed a decreasing trend, the cost of coal- based electricity would drop for a while but eventually be determined by the price of coal, which fluctuates while showing no long-term trend.
- McNerney J, Farmer JD, Trancik JE, Historical Costs of Coal-Fired Electricity and Implications for the Future, Energy Policy, 2011, Vol. 39, pp. 3042-3054 pdf.
Patenting trends: Understanding the factors driving innovation in energy technologies is of critical importance to mitigating climate change and addressing other energy-related global challenges. Low levels of innovation, measured in terms of energy patent filings, were noted in the 1980s and 90s as an issue of concern and were attributed to low investment in public and private research and development (R&D). Here we build a comprehensive global database of energy patents covering the period 1970-2009 which is unique in its temporal and geographical scope. Analysis of the data reveals a recent, marked departure from historical trends. A sharp increase in rates of patenting has occurred over the last decade, particularly in renewable technologies, despite continued low levels of R&D funding. To solve the puzzle of fast innovation despite modest R&D increases we develop a model that explains the nonlinear response observed in the empirical data of technological innovation to various types of investment. The model reveals a regular relationship between patents, R&D funding, and growing markets across technologies, and accurately predicts patenting rates at different stages of technological maturity and market development. We show quantitatively how growing markets have formed a vital complement to public R&D in driving innovative activity; these two forms of investment have each leveraged the effect of the other in driving patenting trends over long periods of time.